Dataframes
With RDataFrame, ROOT offers a modern, high-level interface for analysis of data stored in TTree
, CSV and other data formats, in C++ or Python.
The RDataFrame’s reference guide contains detailed information on ROOT dataframes. Keep reading for a brief introduction to the main concepts. Also see the tutorials for many code examples:
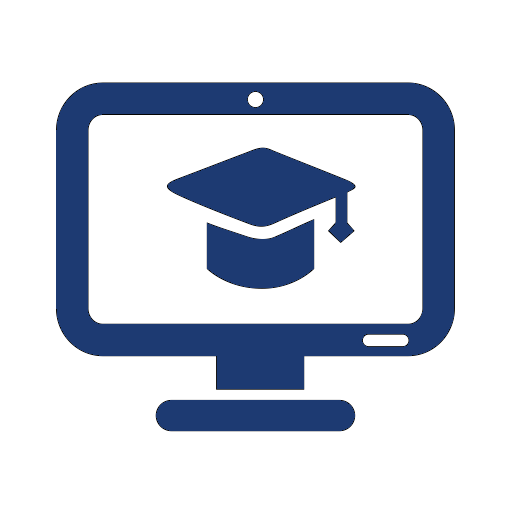
Data analysis with RDataFrame
RDataFrame provides the necessary methods to perform all operations required by your analysis.
Every RDataFrame program follows this workflow:
-
Construct a dataframe object by specifying a dataset. RDataFrame supports single TTrees as well as multiple TTrees (i.e.,
TChain
), CSV files, SQLite files, RNTuples, and it can be extended to custom data formats. From Python, NumPy arrays can be imported into RDataFrame as well. -
Transform the dataframe by:
-
Applying filters. This selects only specific rows of the dataset.
-
Creating custom columns. Custom columns can, for example, contain the results of a computation that must be performed for every row of the dataset.
-
-
Produce results. Actions are used to aggregate data into results. Most actions are lazy, i.e. they are not executed on the spot, but registered with RDataFrame and executed only when a result is accessed for the first time. The most typical result produced by ROOT analyses is a histogram, but RDataFrame supports any kind of data aggregation operation, including writing out new ROOT files.
How does it look in code?
This is a simple cut-and-fill with RDataFrame:
ROOT::RDataFrame df("mytree", {"f1.root", "f2.root"});
auto h = df.Filter("x > 0").Histo1D("x");
h->Draw(); // the event loop is run here, upon first access to one of the results
The lazy triggering of the event loop (i.e. the loop over all data) makes it easy to generate multiple results while reading the data only once:
// C++11 lambda expressions and C++ functions are also supported as filter expressions
auto filtered_df = df.Filter([](float x) { return x > 0; }, {"x"});
auto hx = filtered_df.Histo1D("x");
auto hy = filtered_df.Histo1D("y");
hx->Draw(); // event loop is run here, both hx and hy are filled
In C++, Filter
and Define
expressions can consist of any callable type (e.g. C++11 lambda expressions). Strings containing valid C++ code are also supported, and usually save some typing at a little cost in performance.
As a last example, let’s filter the events, define a new quantity, produce a control plot and write out the filtered dataset, all in the same multi-thread event loop:
ROOT::EnableImplicitMT(); // enable multi-threading (see "Parallel execution" below)
ROOT::RDataFrame df(treename, filenames); // create dataframe
auto df2 = df.Filter("x > 0").Define("y", "x*x"); // filter and define new column
auto control_h = df2.Histo1D("y"); // book filling of a control plot
// write out new dataset. this triggers the event loop and also fills the booked control plot
df2.Snapshot("newtree", "newfile.root", {"x","y"});
Python usage looks very similar. Note that in Python, Filter
s and Define
s require C++ code strings as expressions:
ROOT.EnableImplicitMT()
df = ROOT.RDataFrame(treename, filenames)
df2 = df.Filter("x > 0").Define("y", "x*x")
control_h = df2.Histo1D("y")
df2.Snapshot("newtree", "newfile.root", ("x","y"))
Some Python functions can be injected into RDataFrame thanks to Numba, see this tutorial.
For more examples see the RDataFrame tutorials.
Working with collections
RDataFrame reads collections as the special type RVec: for example, a branch containing an array of floating point numbers can be read as a RVecF. C-style arrays (with variable or static size), STL vectors and most other collection types can be read this way.
RVec is a container similar to std::vector (and can be used just like a std::vector) but it also offers a rich interface to operate on the array elements in a vectorised fashion, similarly to Python’s NumPy arrays.
For example, to fill a histogram with the pt of selected particles for each event, Define
can be used to create a column that contains the desired array elements as follows:
// h is filled with all the elements of `good_pts`, for each event
auto h = df.Define("good_pts", [](const ROOT::RVecF &pt) { return pt[pt > 0]; })
.Histo1D("good_pts");
And in Python:
h = df.Define("good_pts", "pt[pt > 0]").Histo1D("good_pts")
Parallel execution
RDataFrame can perform multi-threaded event loops to speed up the execution of its actions. Each thread will process part of the dataset, and RDataFrame will transparently merge results into the full objects returned to users.
To enable parallel data processing, call the ROOT::EnableImplicitMT() function before constructing a RDataFrame object.
For more information about multi-threading in ROOT, please see Multi-threading.
Experimental distributed execution
It is possible to schedule execution of a RDataFrame application on a computing cluster or other distributed computing resources thanks to the experimental Python package for distributed RDataFrame.
In most cases, no change to an existent Python RDataFrame analysis code is required. For example, the following snippet schedules a simple cut-and-fill task on a Dask cluster:
import ROOT
from dask.distributed import Client
# point RDataFrame calls to the Dask specific RDataFrame
RDataFrame = ROOT.RDF.Experimental.Distributed.Dask.RDataFrame
client = Client("dask_scheduler.domain.com:8786")
# the Dask RDataFrame constructor accepts the Dask Client object as an optional argument
df = RDataFrame("mytree","myfile.root", daskclient=client)
# proceed as usual
sum = df.Filter("x > 10").Sum("y")
h = df.Histo1D("x")
# the RDataFrame event loop is triggered here, upon first access to one of the results,
# and computation runs as Dask tasks
print(sum.GetValue())
Through Dask, computation can be scheduled on a variety of systems, e.g. HTCondor clusters, SLURM clusters or by connecting to computing resources via SSH. Spark clusters are also supported.
Read more on distributed RDataFrame here.